Michael Beetz provides an educational introduction to CRAM, Cognitive Robot Abstract Machine. How can we write a robot control program where the robot receives instructions for the performance of a task and is able to produce the behavior neccessary to accomplish the task? This simple question is not fully answered yet as there is still an information gab between instruction and body motion that has to be filled in a semantically meaningful manner. One way is simplifying perception tasks and implementing motion contrains. Follow Michael Beetz's interesting approach to metacognition.
PhD-Students
Lectures
Part 1: In his first noteworthy lecture, Animesh Garg presents his vision of building intelligent robotic assistants that learn with the same amount of efficiency and generality than humans do through learning algorithms, particularly in robot manipulation. Humans learn through instruction or imitation and can adapt to new situations by drawing from experience. The goal is to have robotic systems recognize new objects in new environments autonomously (diversity) and enable them to do things they were not trained to do by using long-term reasoning (complexity). Animesh Garg introduces the approach to "learning with structured inductive bias and priors", i.e. the ability to generalize beyond the training data.
Part 2: Rachid Alamis' second presentation continues with a dive into the exciting topic of Human-Robot Interaction (HRI). When humans interact with each other, for example by giving a pen to someone else, they exchange (verbal/non-verbal) signals. Rachid Alami gives a very good, short introduction to human-human interaction before exploring the challenges of adopting joint action between humans for human-robot interaction. He presents multiple "decisional ingredients" for interactive autonomous robot assistants.
Part 2: In his second lecture, Kei Okada discusses episodic memory. It describes the collection of past personal experiences in comparison to semantic memory that refers to the general knowledge about the world humans accumulate throughout their life. In order to achieve a goal like tidying up objects, a robot has to rely on acquired knowledge about where to find objects and what to do with them. In a very educational way, Kei Okada further addresses the concepts of task instantiation and experience accumulation mechanism, meaning the formation of patterns and routines in task completion.
Part 1: In the first part of his captivating lecture, Rachid Alami discusses decisional abilities required for Human-Robot Interaction (HRI) and Human-Robot Collaboration in particular. The challenge is to develop and build cognitive and interactive abilities to allow robots to perform collaborative tasks with humans, not for humans. The first part centers on the introduction to Human-Robot Joint Actions and the problems of combining tasks planning (what to do) with motion planning (how to do it), especially for grasping, and how they can be solved.
Tools and Datasets
The video is a tutorial showing the basics of the CRAM framework, which is a toolbox for designing, implementing, and deploying software on autonomous robots. The aim of the tutorial is to (1) give an intuition of what knowledge the robot needs to execute even a simple fetch and place, (2) show how many different things can go wrong and teach writing simple failure handling strategies, and (3) make the user familiar with the API of the actions already implemented in the CRAM framework.
Bio:
Gayane (shortly Gaya) is a PhD student at the Institute for Artificial Intelligence of the University of Bremen. Her main research interests are concentrated in the area of cognition-enabled robot executives. She is currently actively and passionately involved in the development of CRAM. Before joining Michael Beetz's group in November 2013, she worked for one year as a research assistant at Kastanienbaum GmbH (now Franka Emika) with Sami Haddadin, in tight collaboration with the Robotics and Mechatronics Center of DLR. Before that, she has acquired her M.Sc. degree in Informatics with a major in AI and Robotics at the Technical University of Munich. Before coming to Germany, she had a number of short-term jobs in the fields of iPhone game development and web development. She's got her B.Eng. degree in Informatics with a major in Information Security from the State Engineering University of Armenia.
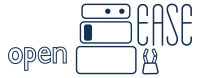
openEASE is a web-based Knowledge Processing Service for Robots and Robotics/AI Researchers.
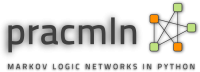
pracmln is a toolbox for statistical relational learning and reasoning and as such also includes tools for standard graphical models. pracmln is a statistical relational learning and reasoning system that supports efficient learning and inference in relational domains. pracmln has started as a fork of the ProbCog toolbox and has been extended by latest developments in learning and reasoning by the Institute for Artificial Intelligence at the University of Bremen, Germany.
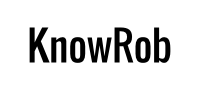
KnowRob is a knowledge processing system that combines knowledge representation and reasoning methods with techniques for acquiring knowledge and for grounding the knowledge in a physical system and can serve as a common semantic framework for integrating information from different sources. KnowRob combines static encyclopedic knowledge, common-sense knowledge, task descriptions, environment models, object information and information about observed actions that has been acquired from various sources (manually axiomatized, derived from observations, or imported from the web). It supports different deterministic and probabilistic reasoning mechanisms, clustering, classification and segmentation methods, and includes query interfaces as well as visualization tools.
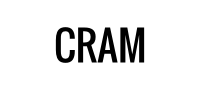
CRAM (Cognitive Robot Abstract Machine) is a software toolbox for the design, the implementation, and the deployment of cognition-enabled autonomous robots performing everyday manipulation activities. CRAM equips autonomous robots with lightweight reasoning mechanisms that can infer control decisions rather than requiring the decisions to be preprogrammed. This way CRAM-programmed autonomous robots are much more flexible, reliable, and general than control programs that lack such cognitive capabilities. CRAM does not require the whole domain to be stated explicitly in an abstract knowledge base. Rather, it grounds symbolic expressions in the knowledge representation into the perception and actuation routines and into the essential data structures of the control programs.